The conversation around artificial intelligence (AI) in data teams is buzzing with excitement—and often, differing levels of expectation. For data leaders, the promise of AI is compelling: automating manual tasks, answering complex questions, and empowering broader access to data insights. But alongside these promises come misconceptions that can distort expectations and hinder successful AI adoption. In this post, we tackle four common myths about AI adoption in data teams, helping you make informed decisions that enhance your team's impact.
Misconception #1: AI Will Replace Data Teams
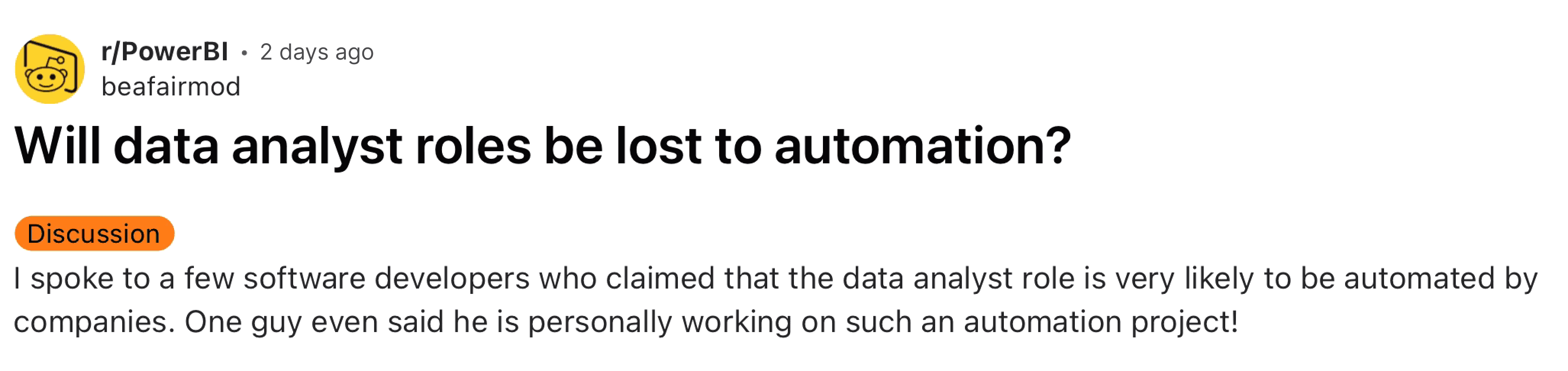
It's easy to fall into the trap of thinking AI will replace data teams. After all, AI can analyse data, generate insights, and answer questions—tasks that might sound similar to what analysts do. However, the reality is far more nuanced. AI is not here to replace data professionals but to augment their capabilities.
Natural Language Querying (NLQ; talking with your data with AI) is a prime example of this. By allowing non-technical users to ask questions in plain language and get answers from their data, NLQ significantly reduces the volume of routine ad hoc requests that data analysts are typically tasked with. Instead of analysts being bogged down by a myriad of minor reporting queries, stakeholders can self-serve for simple data inquiries like, “What was the revenue last quarter for product X?”
With NLQ in place, data teams can shift their focus to high-value, strategic analysis—activities that require critical thinking and domain expertise, areas where AI alone cannot deliver. Rather than displacing data teams, NLQ empowers them to focus on impactful, complex projects while providing accessibility for everyone else.
Misconception #2: AI Implementation is Quick and Easy
The allure of AI often comes with the illusion that implementation is plug-and-play. You simply deploy a model, and it’s off to the races. Unfortunately, real-life AI projects are rarely that simple. Successful AI adoption requires careful planning, data preparation, and integration into existing workflows—none of which happen overnight.
This is especially true for NLQ, which requires an upfront investment to configure the tool so that it understands an organisation’s unique data structures, definitions, and business context.
For example, an NLQ solution needs to be tailored to understand that the term 'customer' can mean different things depending on the department—is it a lead, a subscriber, or someone who has made a purchase? This level of customisation ensures that insights are accurate and relevant. Effective AI implementation requires collaboration among data engineers, analysts, and business users to align configurations with real-world needs.
Misconception #3: AI Can Operate Independently Without Human Oversight
Another common belief is that AI systems, once set up, can operate independently without the need for ongoing maintenance or oversight. In practice, AI—including NLQ solutions—is not a “set it and forget it” technology. Data changes over time, business needs evolve, and new data sources are continuously introduced. As such, NLQ systems require continuous monitoring and updating to ensure they are providing relevant and accurate insights.
Imagine a scenario where new product lines are added, or the company redefines its regional structure. An NLQ system must be updated to reflect these changes. If not, users may receive incomplete or incorrect responses to their questions. Human oversight ensures that the AI continues to deliver high-quality results, staying aligned with evolving business realities.
Data teams play a crucial role in training, monitoring, and refining these systems, maintaining the bridge between business users and reliable data insights. Without it, AI’s will lack any context and hallucinate or ‘guess’ their way to answers.

Misconception #4: AI Solves All Data Problems
AI is a powerful tool, but it’s not a magic bullet that solves all data problems. It’s essential to recognise that AI is only as good as the data it receives and the way it’s used. AI can help identify patterns, provide recommendations, and streamline processes, but it doesn’t replace the need for solid data governance, data quality, and domain expertise.
For example, if there are multiple systems tracking customer interactions without a unified view, an NLQ query like “How many active customers do we have?” might yield conflicting answers depending on the data source it taps into. AI can assist in surfacing insights, but data governance, quality control, and thoughtful integration remain essential tasks for data teams. AI augments data capabilities but doesn’t eliminate the foundational work required to keep data clean and aligned.
For more on how you can train an LLM to perform at its peak, head here.
Embracing to Empower
There are still inevitable misconceptions about AI's role in data teams, leading to unrealistic expectations and sometimes skepticism. AI tools like natural language querying (NLQ) provide significant advancements in data accessibility but are not designed to replace data teams. Instead, NLQ is a tool to democratise data access across an organisation, allowing business users to self-serve while enabling analysts to focus on more complex, strategic work. AI adoption is fundamentally about partnership—between technology and human expertise, between scalability and specificity.
Data leaders can navigate AI adoption more effectively by addressing these kinds of misconceptions. It's an important step to ensuring that tools like NLQ are leveraged to their fullest potential, empowering data teams and business users alike.
Work with Fluent
Put data back into the conversation. Book a demo to see how Fluent can work for you.
29 November 2024
How to build trust with natural language querying
21 November 2024
What's the biggest challenge facing data teams today?
Stay up to date with the Fluent Newsflash
Everything you need to know from the world of AI, BI and Fluent. Hand-delivered (digitally) twice a month.